Introduction
Do you know how always we hear about “diverse” data sets in automatic learning? Well, it turns out there was a problem with that. But don’t worry: A great team of researchers has just dropped a role that changes the game that has the whole ML community. In the article that recently won the ICML 2024 Best Paper Award, researchers Dora Zhao, Jerone Ta Andrews, Orestis Papakyriakopoulos and Alice Xiang address a critical problem in machine learning (ML) – often vague and not declared “diversity claims “In datessets on dates. His work, entitled “measures diversity of data data, not only claims”, proposes a structured approach to conceptualizing, operationalizing and evaluating diversity in ML data sets using principles of measurement theory.
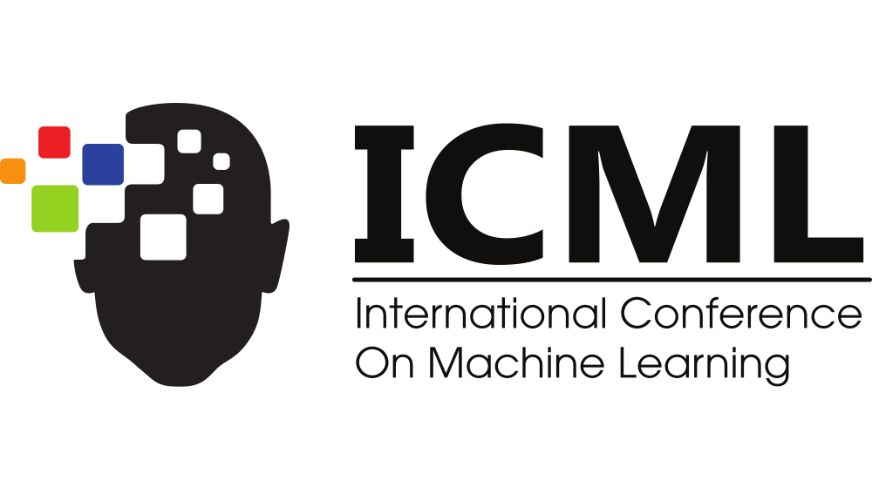
Now, I know what you’re thinking about. “Other jobs on database diversity diversity? Didn’t we hear this before?” But do you trust me, this is different. These researchers made a difficult look at how we used terms such as “diversity”, “quality” and “bias” without backed them. We have been playing fast and loose with these concepts and they are calling us.
But here is the best part: they not only point to the problem. They have developed a solid framework to help us measure and validate claims of diversity. They are handing us a toolbox to solve this messy situation.
So loop because I’m about to take you to a deep dive in this innovative research. Let’s explore how we can go beyond claiming diversity to measure it. Trust me, in the end of this, you will never look at a mL data set in the same way.
The problem of diversity statements
The authors highlight a lost problem in the machine learning community: Data commissioners often use terms such as “diversity”, “bias” and “quality” without clear definitions or validation methods. This lack of precision hinders reproducibility and perpetuates the wrong idea that data sets are neutral entities instead of value -loaded artifacts made up of the social perspectives and contexts of their creators.
A framework for measuring diversity
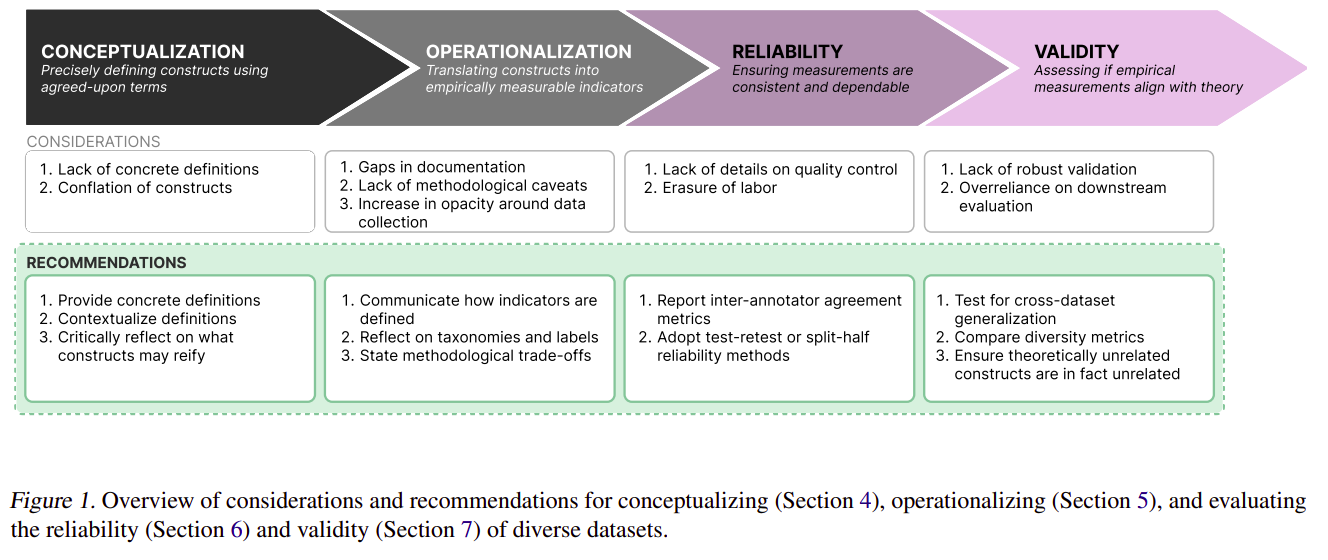
From social sciences, especially measurement theory, researchers present a framework for transforming abstract notions of diversity into measurable buildings. This approach involves three key steps:
- Conceptualization: Clearly define what “diversity” means in the context of a specific data set.
- Operationalization: Develop specific methods to measure the defined aspects of diversity.
- Assessment: Assessment of reliability and validity of diversity measurements.
In summary, this position document defends clearer definitions and stronger validation methods in creating various data sets, proposing the theory of measurement as a scaffolding framework for this process.
Key conclusions and recommendations
Through an analysis of 135 image and text data sets, the authors discovered several important ideas:
- Lack of clear definitions: Only 52.9% of data sets explicitly justified the need for various data. The article highlights the importance of providing specific and contextualized definitions of diversity.
- Documentation gaps: Many jobs that enter data sets do not provide detailed information on collection strategies or methodological options. The authors defend greater transparency in the documentation of the data set.
- Concerns of reliability: Only 56.3% of data sets covered quality control processes. The article recommends using the year-on-year agreement and the reliability of the test-vest to evaluate the consistency of the data set.
- Challenges of validity: Diversity statements often lack robust validation. The authors suggest using techniques based on the validity of construction, such as convergent and discriminating validity, to assess whether data sets truly capture the diversity of planned constructions.
Practical application: the segment of any data set
To illustrate your framework, the article includes a case study case study of any data set (SA-1B). By praising certain aspects of SA-1B’s diversity approach, the authors also identify areas to improve, such as improving transparency around the data collection process and providing stronger validation for geographical diversity claims.
Wider implications
This research has important implications for the ML community:
- Challenge “Thought A Scale”: The article argues against the idea that diversity automatically arises with larger data sets, emphasizing the need for intentional healing.
- Loading the documentation: While advocating for greater transparency, authors recognize the necessary substantial effort and call for systemic changes in the operation of data in ML research.
- Temporary considerations: The article highlights the need to realize how diversity constructions can change over time, affecting the relevance and interpretation of the data set.
You can read the article here: Position: Measure Data Data Data Data, Do not claim it alone
Conclusion
This best ICML 2024 role offers a path to more rigorous, transparent and reproducible research by applying principles of measurement theory to ML data data creation. As the field grabs with bias and representation issues, the framework presented here provides valuable tools to ensure that diversity claims in ML data sets are not just rhetorical but measurable and significant contributions to developing fair and robust systems.
This innovative work serves as a call for action so that the ML community raises the healing and documentation standards of the data set, which leads to learning models of more reliable and equitable machines.
I have to admit that when I first saw the recommendations of the authors to document and validate the data sets, a part of me thought, “Ugh, that seems a lot of work.” And yes, that’s the case. But you know what? It is work that needs to be done. We cannot continue to build AI systems in trembling foundations and we just look forward to the best. But this is what shot me: this article is not just about improving our data sets. It is about doing our entire field more rigorous, transparent and trustworthy. In a world where IA is becoming more and more influential, that’s huge.
So what do you think? Are you ready to wrap the sleeves and start measuring data diversity? Let’s talk in the comments: I would love to hear your thoughts about this research that changes play.
You can read another ICML 2024 best paperHere is here: ICML 2024 Top Papers: News in machine learning.
Frequent questions
Anx. The diversity measurement of the database is crucial because it ensures that the data sets used to train machine learning models represent various scenarios and demography. This helps reduce biases, improve the generalization of models, and promote equity and equity in AI systems.
Anx. Various data sets can improve the performance of ML models by exposing them to a wide range of scenarios and reducing adaptation to any particular group or scenario. This leads to more robust and precise models that work well in different populations and conditions.
Anx. Common challenges include defining what is for diversity, operating these definitions in measurable constructions, and validating claims of diversity. In addition, ensuring transparency and reproducibility to document the diversity of data sets can be intensive in work and complex.
Anx. Practical steps include:
A. Define clearly specific goals and criteria of diversity for the project.
b. Collecting data from various sources to cover different demographics and scenarios.
c. Using standardized methods to measure and document diversity in data sets.
d. Continuously evaluate and update data sets to maintain diversity over time.
E.Implicating robust validation techniques to ensure that the data sets really reflect the expected diversity.